AI tokens serve as the fundamental units of computation in artificial intelligence, encompassing words, subwords, and non-textual elements across modalities. They function as both the currency of machine cognition and the building blocks that enable models to process information efficiently. Beyond text, tokenization extends to images, audio, and video, with economic implications for processing costs and user experience. Understanding tokens reveals the true mechanics behind AI’s seemingly magical capabilities.
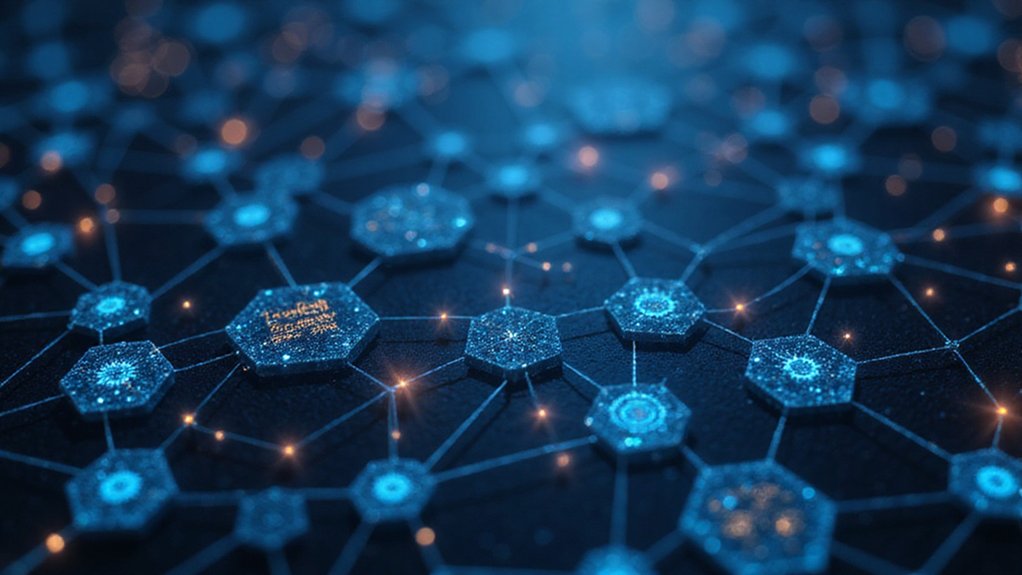
The labyrinthine world of artificial intelligence operates on a foundation that remains invisible to most users: tokens, the atomic units that fuel every AI system’s ability to process, understand, and generate information.
These fundamental components serve as the currency of computational cognition, allowing algorithms to dissect and manipulate information with remarkable dexterity.
While often conceptualized merely as words, AI tokens transcend linguistic boundaries, encompassing subwords, characters, and even non-textual elements depending on the specific tokenization methodology employed.
AI tokens extend beyond simple words, embracing fragments, characters, and non-verbal components across diverse tokenization architectures.
The tokenization process—that critical conversion of raw data into digestible units—varies dramatically across model architectures.
Sophisticated systems leverage subword tokenization to decompose unfamiliar terminology into recognizable fragments, thereby expanding their effective vocabulary without corresponding increases in computational requirements.
This elegant solution (one might say an arbitrage of linguistic efficiency) allows models to navigate the treacherous waters of novel words, rare terminology, and cross-linguistic patterns that would otherwise render them functionally illiterate.
Beyond the textual domain, modern AI systems apply tokenization principles across modalities.
Images become grids of pixel tokens, audio transforms into sequential sound frames, and video resolves into temporal-spatial token sequences—all processed through increasingly unified computational frameworks.
This multi-modal approach has catalyzed the development of systems capable of reasoning across traditionally siloed information types.
The economic implications of token processing efficiency cannot be overstated.
During inference processes, models balance time constraints through metrics like time to first token and subsequent generation rates, directly impacting user experience.
Context length capabilities represent a crucial aspect of token utilization, directly affecting an AI model’s ability to maintain coherence in responses across extended interactions.
As processing costs scale with token volume, optimization techniques that reduce token requirements or accelerate their processing represent direct financial value.
Indeed, the marketplace increasingly recognizes that an AI system’s worth correlates directly with its token throughput capabilities.
In parallel to computational tokens, the emergence of AI-specific cryptocurrencies has created additional complexity in the ecosystem.
Platforms like Bittensor have emerged to democratize AI development through decentralized markets where machine learning models can be developed, trained, and traded using blockchain technology.
These blockchain-based assets, often structured as ERC-20 tokens, provide governance rights and access privileges within decentralized AI platforms—effectively tokenizing the tokenization process itself.
Such meta-financial instruments represent the convergence of distributed ledger technology with computational linguistics, creating novel economic structures around AI resource allocation.
Frequently Asked Questions
What Are the Largest AI Tokens by Market Capitalization?
The largest AI tokens by market capitalization currently include Fetch.ai (FET) with its billion-dollar valuation and token price around $0.57, TAO Network commanding an impressive $296.38 per token, and DCK (DeepBrain Chain) at $0.0095.
NEAR Protocol, while not exclusively AI-focused, ranks prominently in the sector as well.
The entire AI token ecosystem represents approximately $22.2 billion in market cap, with numerous smaller players like Cudos and Assemble AI rounding out the landscape.
Can AI Tokens Be Mined Like Bitcoin?
Most AI tokens don’t employ Bitcoin’s energy-intensive Proof of Work mining. Instead, they utilize alternative acquisition methods—staking (Ocean Protocol), contributing computing resources (Fetch.ai), running AI nodes (SingularityNET), or providing valuable datasets.
These mechanisms represent a fascinating pivot from raw hash power to utility-driven participation.
While technically not “mining” in the traditional sense, these contribution-based earning systems serve analogous economic functions, though with dramatically different resource requirements and environmental footprints.
How Do AI Tokens Impact Blockchain Energy Consumption?
AI tokens impact blockchain energy consumption through algorithmic efficiency improvements that traditional cryptocurrencies sorely lack.
By implementing predictive modeling and optimization techniques, these tokens facilitate more energy-efficient consensus mechanisms—particularly the shift from power-hungry Proof-of-Work to Proof-of-Stake systems.
The environmental benefits are substantial; case in point: Ethereum’s shift to PoS slashed energy requirements by approximately 99.95% (a figure that should give Bitcoin maximalists pause).
AI-driven validation substantially reduces computational demands, lowering both carbon footprints and transaction costs.
Are AI Tokens Regulated Differently Than Other Cryptocurrencies?
AI tokens largely face the same regulatory frameworks as other cryptocurrencies, with nuanced distinctions emerging from their utility functions.
While both operate under blockchain-based AML/KYC requirements, AI tokens‘ dual nature as service-access mechanisms and governance instruments often triggers additional regulatory considerations.
Their integration with AI systems potentially invokes data privacy regulations that purely transactional cryptocurrencies might avoid.
Jurisdictional approaches vary considerably, though most regulators are adapting existing frameworks rather than crafting AI token-specific legislation.
What Investment Risks Are Specific to AI Tokens?
AI tokens carry unique investment risks beyond typical cryptocurrency concerns.
These include algorithmic vulnerabilities (what happens when the underlying AI becomes obsolete?), heightened volatility from speculative trading in nascent technology, and distinctive regulatory uncertainty as governments grapple with dual-layer complexity.
Furthermore, investors face exposure to both the token’s blockchain infrastructure risks and the AI system’s operational challenges—a precarious double-dependency that can amplify market fluctuations when either component falters.
Data integrity issues further compound these specialized risks.