Black Box AI epitomizes modern tech’s central paradox: algorithms transforming society while remaining fundamentally inscrutable. These systems—from facial recognition to hiring algorithms—operate through neural networks so complex that even their creators can’t fully explain outputs. Opacity emerges both intentionally (protecting IP) and inevitably (computational complexity), raising accountability concerns in critical sectors like healthcare and finance. The tension between advancement and comprehensibility has spawned Explainable AI initiatives, though cracking these algorithmic enigmas proves no simple feat.
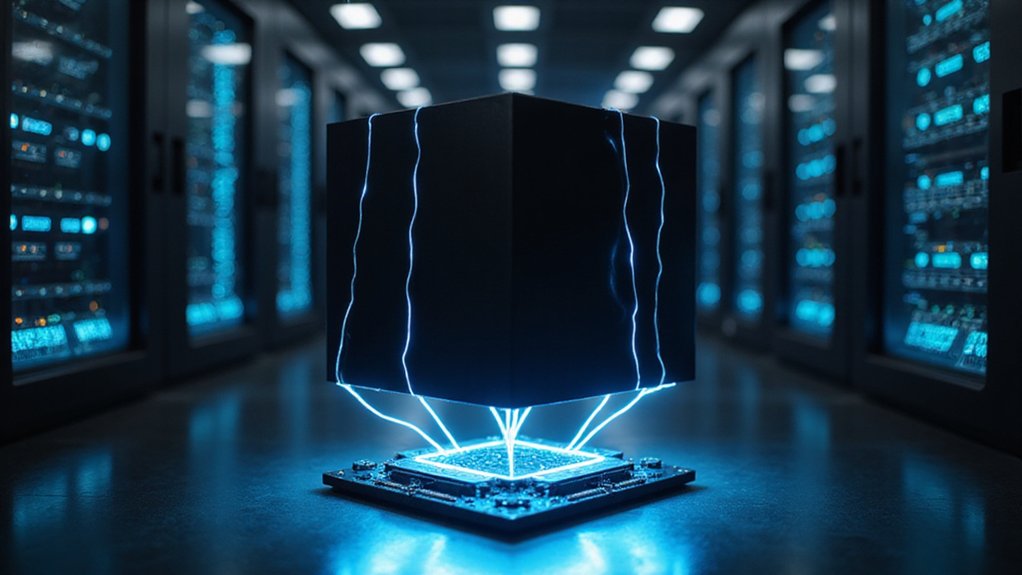
The enigmatic world of “black box AI” represents perhaps the ultimate paradox in modern technology: systems of unprecedented power and utility whose inner workings remain stubbornly inscrutable to human understanding.
These sophisticated systems—particularly deep learning models with their labyrinthine neural networks—produce outputs while keeping their decision-making processes hidden behind an impenetrable veil.
The visible inputs and outputs frame a mysterious processing core, creating what amounts to a high-stakes computational magic trick performed daily across global markets and platforms.
This opacity manifests through both intentional and emergent characteristics.
The veil of algorithmic secrecy exists both by design and as an inevitable consequence of computational complexity.
Proprietary algorithms deliberately shroud their source code to protect intellectual assets, while deep learning models naturally evolve into black boxes as their multilayered neural networks—often comprising hundreds to thousands of layers—disperse decision logic beyond comprehension.
Even their creators frequently cannot fully explicate the intricate dance occurring among tens of thousands of artificial neurons processing unstructured data at unprecedented scale.
These inscrutable systems have nonetheless become ubiquitous fixtures in daily life.
Emerging platforms like Bittensor network aim to democratize access to AI by creating decentralized ecosystems that potentially make black box algorithms more transparent and accessible beyond corporate control.
Facial recognition accessing smartphones, Alexa interpreting natural language commands, ChatGPT generating human-like text, and hiring algorithms silently evaluating job applicants—all leverage black box architectures with minimal transparency regarding their internal calculations.
The consumer’s experience, much like an iceberg, reveals only the polished interface floating above a vast computational infrastructure.
Leading models like OpenAI’s ChatGPT exemplify the black box problem, delivering impressive results while concealing their internal mechanisms from scrutiny.
The financial and ethical implications of this technologically-induced opacity cannot be overstated.
How does one assess accountability when neither user nor developer can articulate the reasoning behind a credit denial or insurance premium calculation?
The debugging and validation processes become exercises in educated guesswork rather than systematic analysis.
These systems raise serious concerns across critical sectors like healthcare and finance, where decisions made through complex neural networks can significantly impact human lives without providing explanations.
These challenges have spawned a counter-movement in the form of Explainable AI (XAI), with techniques like LIME attempting to pry open these algorithmic black boxes.
The tension between technological advancement and transparency creates a peculiar inflection point in our digital evolution—systems simultaneously becoming more capable and less comprehensible, raising profound questions about governance, trust, and the appropriate boundaries of algorithmic decision-making in an increasingly automated world.
Frequently Asked Questions
How Does Black Box AI Impact Personal Privacy?
Black box AI compromises personal privacy through multiple vectors: its opacity prevents individuals from knowing how their data is processed or used; hidden biases may result in discriminatory profiling; security vulnerabilities enable unauthorized access to sensitive information; and the inability to audit these systems complicates regulatory compliance.
This technological enigma—impenetrable to scrutiny yet privy to intimate details—creates a privacy paradox where individuals surrender data to systems whose inner workings remain deliberately obscured from view.
Can Black Box AI Systems Be Fully Ethical?
Fully ethical black box AI systems remain an elusive ideal, given their inherent opacity challenges.
While technological solutions like XAI and algorithmic auditing represent promising steps forward, the fundamental tension between proprietary complexity and ethical transparency persists.
The implementation of robust oversight mechanisms, diverse training data, and thorough regulatory frameworks may narrow this ethics gap, but perfection seems improbable when decisions affecting human lives emanate from processes that, by definition, resist complete scrutiny—a Faustian bargain of efficiency versus accountability.
What Skills Are Needed for Careers in Explainable AI?
Careers in explainable AI demand a multifaceted skillset that marries technical prowess with ethical sensibilities.
Professionals must master machine learning algorithms, programming languages (Python reigns supreme), and specialized tools like LIME and SHAP—the financial derivatives of the AI explanation market, if you will.
Statistical acumen, data visualization capabilities, and regulatory knowledge (GDPR, anyone?) form the backbone of expertise, while communication skills—that rare ability to translate algorithmic hieroglyphics into stakeholder-palatable narratives—complete the package.
How Do Governments Regulate Black Box AI Internationally?
Governments regulate black box AI through a patchwork of approaches featuring risk-based frameworks that prioritize oversight of high-consequence applications.
The EU leads with its thorough AI Act, while international bodies like the OECD and G20 attempt to establish interoperable standards across jurisdictions.
Transparency requirements—compelling developers to document training data and decision processes—form the regulatory backbone, though enforcement mechanisms remain inconsistent.
The jurisdictional challenges mirror earlier internet regulation struggles, with sovereignty issues complicating coherent global governance.
Will Black Box AI Eventually Become Completely Transparent?
Complete transparency in black box AI remains improbable despite ongoing advances in explainable AI technologies.
The fundamental trade-off between performance and interpretability suggests a more realistic outcome: tiered transparency where critical decision-making systems face stricter disclosure requirements than consumer applications.
Technical barriers notwithstanding, market forces (where opacity often confers competitive advantage) will likely guarantee that—much like proprietary trading algorithms—certain AI systems will maintain their enigmatic core while offering selective transparency where absolutely necessary.